TrustAxis is a global health alliance with the goal of fostering collaborative healthcare innovation by connecting researchers and innovators with health datasets and compute facilities through a trusted collaborative corridor. The vision behind creating TrustAxis is to achieve impactful results in global healthcare innovation. The need for data collaboration arises from challenges such as addressing data silos, navigating data regulatory and sharing policies, ensuring security, and overcoming the difficulty of transferring large quantities of data across national boundaries. TrustAxis aims to establish communities of practice based on prioritized themes. These communities would likely consist of experts, researchers, and innovators who share common interests and goals in healthcare innovation.
The objectives of the alliance are:
- To conceptualize, build, deploy and manage the trusted collaborative corridor infrastructure.
- Build and advocate communities of practice based on prioritized themes.
- Build capabilities to homogenize data of custodians who are at different levels of data maturity.
Transformative AI-led innovations in Healthcare requires access to data, compute, and relevant tools. Access to these resources is constrained by several challenges. Healthcare data ends up in organizational silos due to regulatory frameworks like GDPR and HIPAA, large size of the data preventing data transfer, and unknown value of the data in an AI-led data economy etc. Similarly, compute resources and tools require significant upfront investments which is beyond the reach of most researchers around the world, particularly in LMIC countries.
The key components of TrustAxis are shown in the figure below:
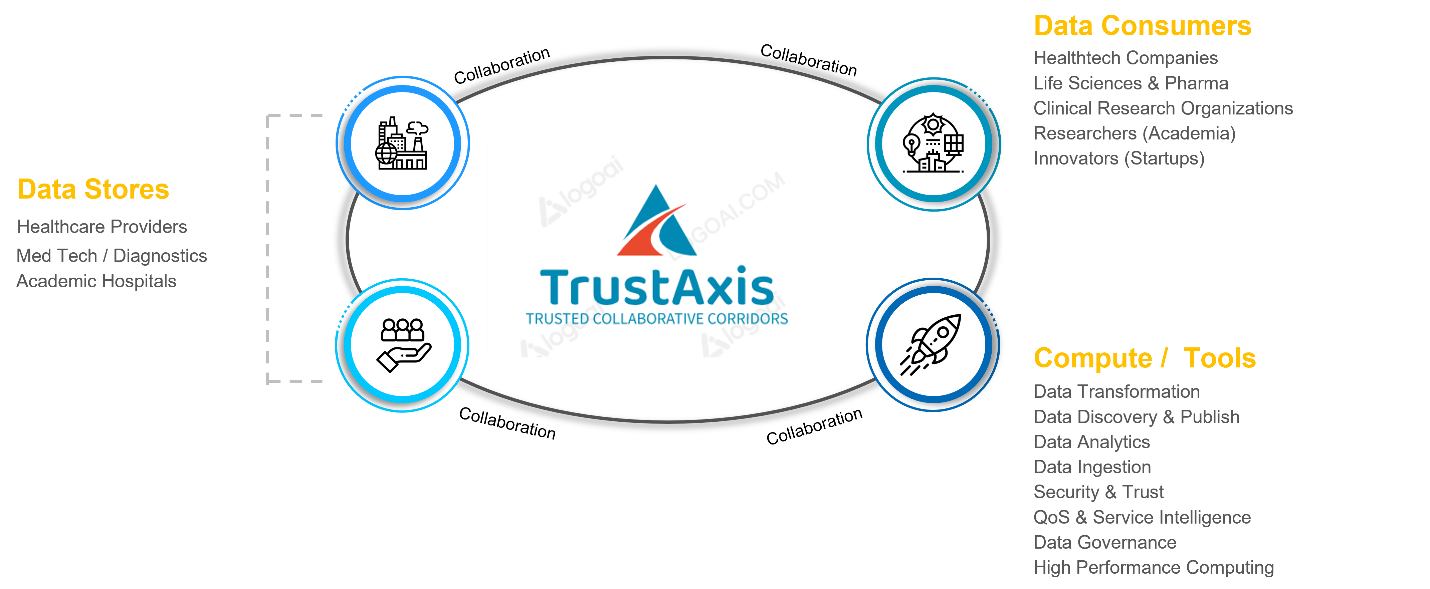
The alliance targets to create a data consortium of Healthcare providers, Med Tech/Diagnostics, Academic hospitals, healthcare data available from government efforts, etc. with the intent / objective to create a data centric “environment for innovation” in healthcare technology. The consortium will provide a secure data collaboration infrastructure including methodologies like Secure Federated Learning to enable the member organizations to get access to quality health data sets from the data consortium irrespective of the physical geographical boundaries, be compliant to applicable regulatory requirements, harness the power of applicable underlying hardware and software platform enabling them to collaborate in a more enhanced secure environment and without imposing any mandatory requirement to physically move the data across legal entities or borders. The alliance will provide access to rich compute and tooling resources through its own investments as well as by enabling access to such resources made available by the participating member. The infrastructure will provide methodologies and mechanisms to bring together the disparate sources of data, compute, and tools to enable a collaborative environment for healthcare innovation.
Impact
The larger impact of this alliance will be to reduce the cost of care, improve the quality of care and increase access to affordable healthcare by reducing the cost of research.
- Builds Trust - The framework enables secure mechanisms by which health researchers can have access to health data, compute, and tools from across different legal entities and across geographies without any concerns on intellectual property breaches. This will help cultivate and promote trust amongst the beneficiaries to participate in transformative research without concerns of breach of their intellectual property assets.
- Reduces costs and promotes faster research outcomes on prioritized themes – Trust helps speed up transformative research as the barriers to access data, compute, and tools is reduced. Faster research translates to lower cost of research. The framework will ensure that the data is in a standardized structure across the collaborative setup which helps speed up the process of research by making available diverse data types based on therapeutic areas such as Radiology, Clinical, Pathology, Genomic data etc.
- Improves quality of care - AI models that otherwise would need to be retrained in each geography separately can now securely get access to data across geographies to ensure high accuracy, repeatability and reproducibility levels enabling them meeting the screening or diagnosis regulatory requirements.