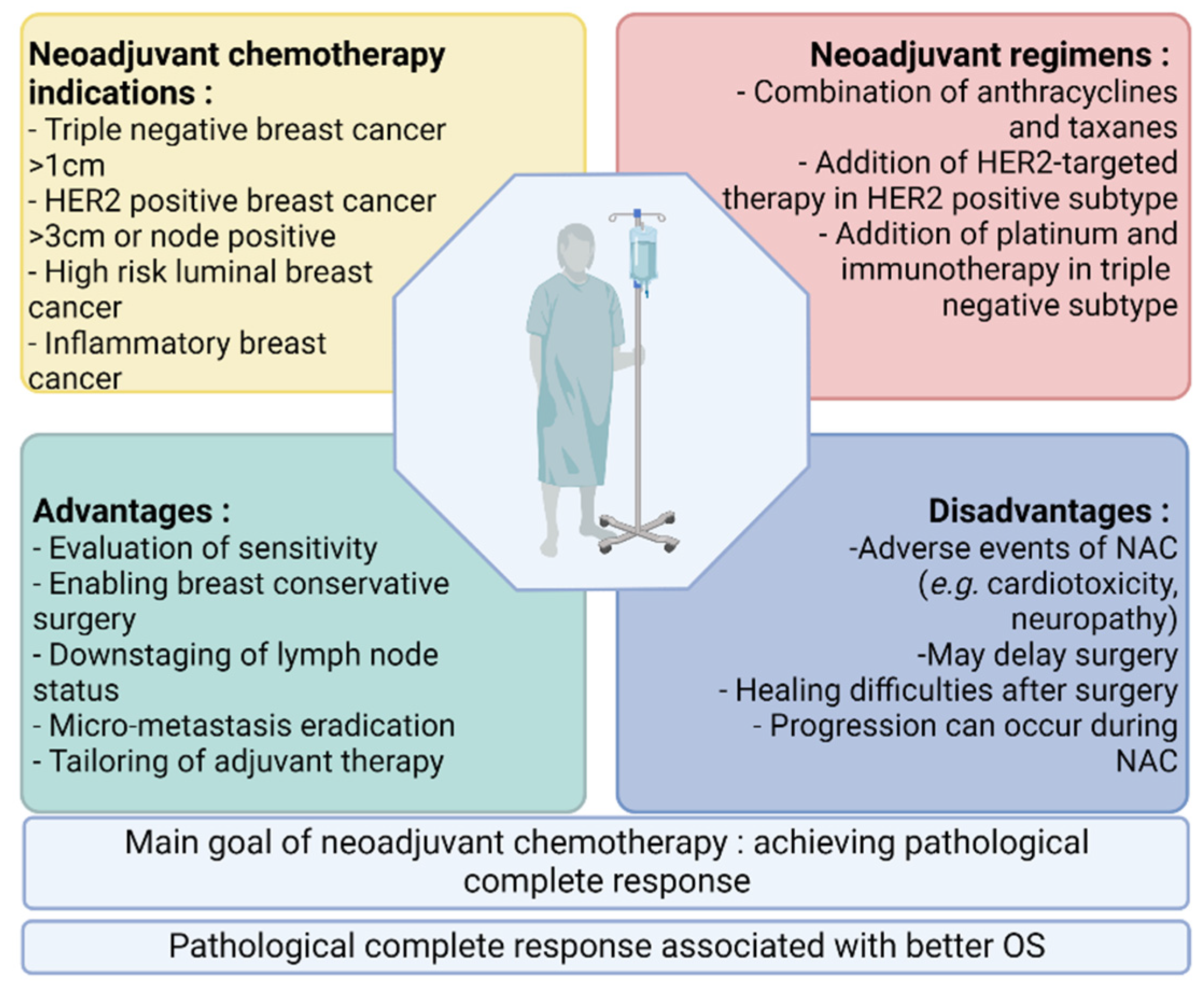
What's the project about?
The project is about the side effects a drug can cause in patients with different diseases and cancers. The half-life of the drug changes as the interaction of the drug changes in different atmospheres and different compositions of cells. This project aimed to identify these effects on drugs and their doses. In this project, we want to cover different types of cancer and the therapies and drugs we use to treat the patients.
What's the goal of the project?
The predictive tool for cancer therapy side effects uses co-morbidities and polypharmacy data to forecast potential adverse reactions in patients undergoing cancer treatment. By analyzing the patients' existing medical conditions (co-morbidities) and the number of medications they are taking (polypharmacy), the tool aims to identify high-risk individuals who may experience heightened side effects during cancer therapy.
What's the outcome expected?
The expected outcome of the predictive tool for side effects of cancer therapy based on co-morbidities and polypharmacy is to improve patient care and treatment outcomes. By identifying high-risk patients who may be more susceptible to side effects due to their pre-existing medical conditions and multiple medications, healthcare professionals can take proactive measures to mitigate potential adverse reactions. This could include adjusting the cancer treatment plan, providing additional support, or closely monitoring patients during therapy. Ultimately, the tool aims to enhance patient safety, reduce the occurrence and severity of side effects, and optimize the effectiveness of cancer treatment, leading to better overall health and quality of life for cancer patients.
Plan of Action (summary)
Our taken course of action involves comprehensive research and data collection on drug side effects in patients with different diseases and cancers, focusing on drug interactions and their impact on half-life. Using this data, a predictive tool will be developed to forecast potential adverse reactions during cancer treatment, considering co-morbidities and polypharmacy. The tool's accuracy will be validated through testing and integrated into healthcare systems for seamless usage. Medical staff will be trained to utilize the tool, offering personalized care to high-risk patients, and continuous improvement will be ensured by updating the model with new data. The tool's impact will be assessed, and findings will be shared with the medical community to enhance patient care and optimize cancer treatment outcomes.